The Role of Machine Learning in Azure AD User Monitoring. In our rapidly evolving digital landscape, the traditional concept of an “endpoint” has transcended its old definition of merely being a user’s device. With the widespread integration of cloud-based identity providers, user directories are no longer confined within the secure boundaries of network perimeters. This paradigm shift has nudged organizations towards adopting zero-trust models, recognizing that identities and Software as a Service (SaaS) platforms are now accessible externally.
The challenge of maintaining complete visibility is increasingly daunting for many. Security teams are overwhelmed with a constant stream of alerts, while also needing to comprehend the diverse ways in which their users access services from various locations. Additionally, they are required to stay updated with the latest attack methods and indicators of attack (IOA) to effectively identify malicious activities. This is an overwhelming task, seemingly too vast for any workforce to handle effectively. The pressing question then becomes: What measures can be implemented to manage this situation?
Introducing Machine Learning in Azure AD Monitoring
Machine learning extends beyond the realm of security, yet its impact in this field has been widely seen. Companies like Microsoft are channeling vast amounts of telemetry data into machine learning models, enabling them to identify anomalies. An example solution would be Microsoft’s Entra ID Protection, which shows how machine learning is revolutionizing security measures.
The picture above should show you the scale of data these models are learning from. Without large organizations like Microsoft, utilizing machine learning, this would not be possible. Remember, they are not only ingesting large amounts of data, but also processing and training the models from it.
The most impressive aspect of this, is that it’s done in near real time. This is often overlooked as we take for granted just how quickly solutions such as these identify, alert and often remediate risk in a matter of milliseconds.
Entra ID Protection
Entra Identity Protection, uses sophisticated machine learning algorithms to safeguard against identity-related threats. It’s continuously ingesting and analyses a vast amount of telemetry data from your Entra ID instance. This data includes user sign-in behaviour, device types, location information, and access patterns.
The machine learning models used in Entra are trained on a broad set of data. That enables them to identify subtle and complex threat patterns. Well, these models are constantly updated by Microsoft to adapt to the evolving landscape of cyber threats. This continuous learning process is vital for maintaining the effectiveness of threat detection mechanisms.
When a potential threat is detected, Entra ID generates alerts. A deep analysis carried out by the machine learning models. Each alert is categorized based on the type of threat detected, such as sign-in risks, user risks, or investigation alerts. The types of alerts and their definitions are meticulously maintained by Microsoft in their documentation, as mentioned in the provided link.
Moreover, the response to these alerts is crucial. Microsoft provides guidance on how to address each type of alert, aiding administrators in taking appropriate action. This could involve initiating automated responses, enforcing conditional access policies, or manually intervening to mitigate the threat.
Entra ID License Consideration
Importantly, the efficacy of these alerts depends on the license tier of Entra ID being used. Microsoft offers 2 options. Each tier provides varying levels of protection, with more advanced features, including sophisticated machine learning-driven threat detection, available in higher-tier licenses (P2).
Read more here: https://learn.microsoft.com/en-gb/entra/id-protection/overview-identity-protection#license-requirements
Try our Active Directory & Office 365 Reporting & Auditing Tools
Try us out for Free. 100’s of report templates available. Easily customise your own reports on AD, Azure AD & Office 355.
The Role Of Machine Learning
Welcome to the age of machine learning. Whilst humans can’t keep track of every user’s telemetry, a trained model can. Microsoft understands this and brings AI and machine learning into the heart of its security platforms. Microsoft trains these models to understand patterns, and flag anomalies but that’s not all.
To reduce false positives, the trained model is able to baseline each detection against risk and a variety of factors. Examples: past events, the device in question, the reputation of the IP source, the source country, the application accessed, the time of day and many more. All of this in seconds.
The quicker an organisation is to react, the less damage an attack can do. Whilst it may be possible for humans to spot these, it’s far quicker to let the machines.
Look at the dashboard above, to show an example of the scale of protection: Microsoft Entra ID Protection overview preview – Microsoft Entra ID Protection | Microsoft Learn
Different Types Of Alerts in Microsoft Identity Protection
Microsoft Identity Protection has various alerts based on different use cases. Some examples:
- Sign-In Risk Alerts: Generated when a sign-in attempt appears to be risky. This could be due to the sign-in originating from an anonymous IP address, an unfamiliar location, or showing patterns typical of a brute force attack.
- Leaked Credentials Alerts: Triggered when a user’s credentials have been detected on the dark web or in other external sources.
- Impossible Travel to Atypical Locations: Generated when there are sign-ins from locations at a distance from each other where travel in the time between sign-ins is impossible.
- Anonymous IP Address Alerts: Triggered when a sign-in occurs from an IP address known to be associated with anonymous proxy services. Often used by attackers to hide their true location.
- Unfamiliar Sign-in Properties: Generated when a sign-in occurs with properties (like device or location) that are unfamiliar based on the user’s past behaviour.
- Malware Linked IP Address Alerts: Raised when sign-ins come from IP addresses known to be associated with malware activity.
Microsoft Identity Protection uses machine learning algorithms and heuristic rules to generate these alerts. They are then investigated and mitigated by cybersecurity teams. The system’s capabilities are continuously evolving, and it’s integrated with other Microsoft security products to provide a comprehensive security posture.
Read more: https://learn.microsoft.com/en-us/entra/id-protection/concept-identity-protection-risks
Thank you for reading our article The Role of Machine Learning in Azure AD User Monitoring. We hope it was enjoyable.
The Role of Machine Learning in Azure AD User Monitoring Conclusion
Because Microsofts telemetry is often unmatched, these models are constantly trained on the latest attack methods. That means you can be pretty confident that the solutions or models are on level, or if not one step ahead of attackers.
Thankfully Microsoft continues to embed machine learning and AI and for those wanting to keep in the know, should visit https://techcommunity.microsoft.com/t5/ai-machine-learning-blog/bg-p/MachineLearningBlog
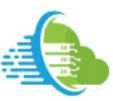
Try InfraSOS for FREE
Try InfraSOS Active Directory, Azure AD & Office 365 Reporting & Auditing Tool
- Free 15-Days Trial
- SaaS AD Reporting & Auditing Solution
Related posts:
- Azure AD Identity Protection: Detect & Respond to Identity Threats
- Using Azure AD Identity Protection to Monitor User Activity
- Windows Server Patch Management: How to Keep Windows Server Secure & Up-to-Date
- How Password Hash Synchronization Works with Azure AD Connect
- Create Active Directory Security Reports with PowerShell